Data Enablement for Regulated Industries
Transparent, explainable AI for high-impact, low-risk environments.
What we do
Fluence is an advanced analytics company.
We deliver auditable, data-driven business transformation for regulated industries.
Our uniquely auditable Machine Learning framework empowers organizations in regulated sectors to harness the power of Artificial Intelligence with confidence and strong data governance.
By fostering a perfect understanding of organizational data and its impact on performance, we enable our clients to maximize sales, optimize resources, and manage risk.
Through our comprehensive approach and tailored solutions, we are a trusted data partner for regulated organizations worldwide, driving their continuous growth and success.
“Partnering with Fluence on a machine learning initiative helped us uncover the unexplored depths within our data and the extensive scope to enhance our work procedures.
The outcomes exceeded our expectations, even enabling us to foresee the impact of specific market activities. This newfound ability allows us to concentrate our efforts on only the most efficient activities when promoting products.
The strong impact we have seen in the pilot led us to continue on this promising venture. We are now transitioning from the pilot phase to an expansive implementation, a step that promises to enhance our efficiency and performance even more.”
Daniel Imwinkelried – IFT Excellence Manager
AbbVie Switzerland
Alte Steinhauserstrasse 14
CH – 6330 Cham
Maximize Desirable
Outcomes
Align activity with company performance.
Sales & Marketing Optimization
Data-driven Investment
Launch Strategies
Optimize Existing Resources
Get the most out of existing tools, processes and teams.
Intelligent Automation
Process Optimization
Business Transformation
Minimize Losses and Risks
Preempt risks. Adapt activities to minimize exposure to losses.
Quality Control
Risk Management
Regulatory Compliance
AI re-designed for clarity and impact
At Fluence, we have re-imagined how Machine Learning trains on data.
Following years of research and development, our team has produced a Machine Learning Framework designed to expose causal relationships between the data and features in the model and their relative impact on complex commercial outcomes.
Our algorithms allow companies to distill large volumes of unstructured data, both internal and external, in free text or structured formats, to provide data-driven answers to burning commercial questions.
Not only do we allow clients to predict insights and monitor performance, our models produce useful feedback on how the models were shaped and how decisions were made. This gives our clients the power to use their data proactively, uncover new patterns, and improve their business strategies.
The technology has been deployed in a range of settings, from automated analytics, predicting future outcomes, and generating or editing content to maximize probability of success.
Competencies
Benefits
- Quantify the impact of unstructured activity on core performance.
- Identify the key drivers of organizational performance and how to measure them.
- Validate and substantiate commercial assumptions.
- Maximize the effectiveness and efficiency of existing resources.
- Bring teams into closer connection with their data.
- Capture and disseminate best practice across teams.
- Bring targets and KPIs into alignment with optimal business outcomes.
Maximizing sales and marketing impact:
We have helped clients develop a deeper understanding on the relationship between sales activity and business performance.
Key Questions Answered:
Which investments, activities and communications maximize the sales potential of the product?
What is the most effective and efficient use of sales and marketing resources?
What is the optimal sequencing of activities, moving prospects through a complex journey from education to patient initiations?
What are the best ways to measure and monitor campaign performance/impact?
What are the best ways of adapting content and sales activities to maximize engagement and conversion?
What is the best way of hyper-personalizing communications to maximize engagement and conversion?
What best practice can be disseminated between teams, countries and brands?
Analytics that work for you
Organic Analytics
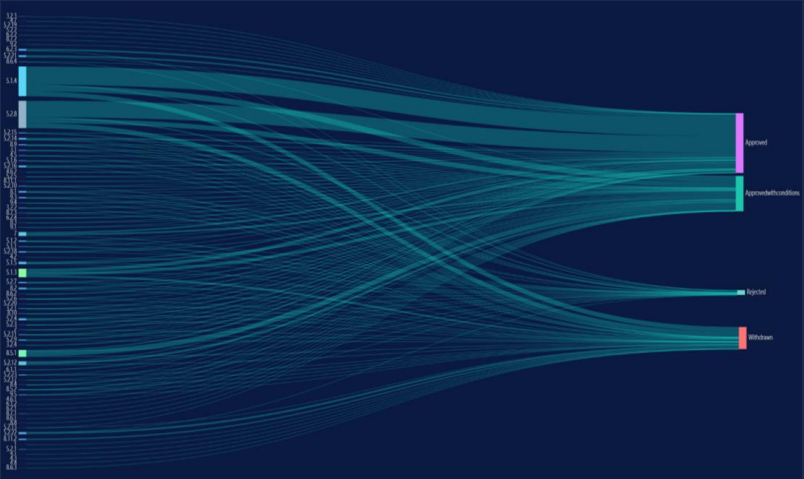
Explore natural patterns in your data and how they align to your commercial outcomes.
Identify patterns of activity associated with specific commercial outcomes, or that are prone to elevated risk or reward.
User-led Analytics
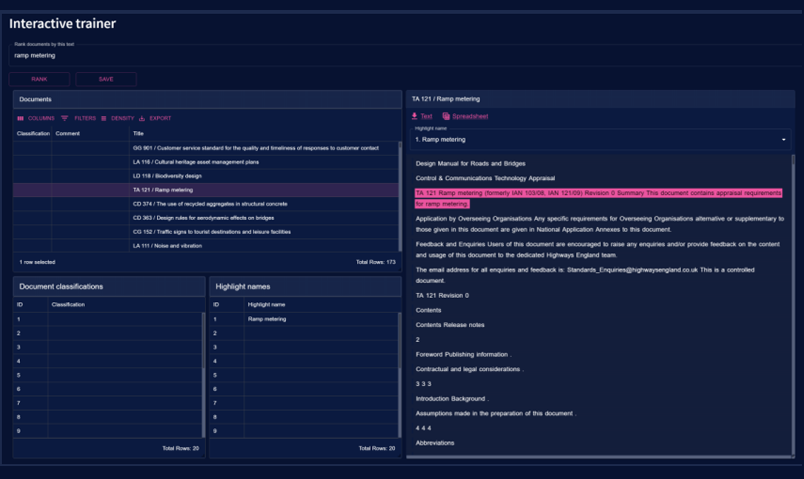
Capture, standardize and harmonize the views of multiple experts.
Users can impart their expertise onto documents, allowing Fluence to harness their knowledge, combine it with other experts, and monitor decision variance between experts.
Performance-led Analytics

Identify patterns of activity that are directly correlated to top-level company performance.
Connect your content and unstructured data to real-world performance metrics (e.g. sales volumes, financial performance, or safety logs) to surface valuable commercial optimizations.
About Fluence
Founded in 2016, Fluence is a British/Swiss deep-tech Al company, bringing together some of the finest engineers from Cambridge University and ETH Zurich. The team has decades of senior engineering experience at major Silicon Valley companies.
We are dedicated to the development and refinement of our flagship product, the Fluence Engine. This robust Machine Learning framework serves as the backbone of our client-facing solutions, enabling us to deliver exceptional performance across various industries.
Its versatility makes it industry-agnostic, proving its effectiveness in domains ranging from education management to pharmaceutical product launches. Rigorous stress-testing has solidified the Fluence Engine’s reliability and efficiency, ensuring it meets and exceeds the highest standards of data management and process governance.
“A disconnect exists between what sales and marketing teams do, and what Healthcare Professionals want to know. This is an issue that Fluence allows us to address.”
Danny Donkers – General Manager
Amgen